Nvidia Dominates Latest MLPerf AI Benchmarks
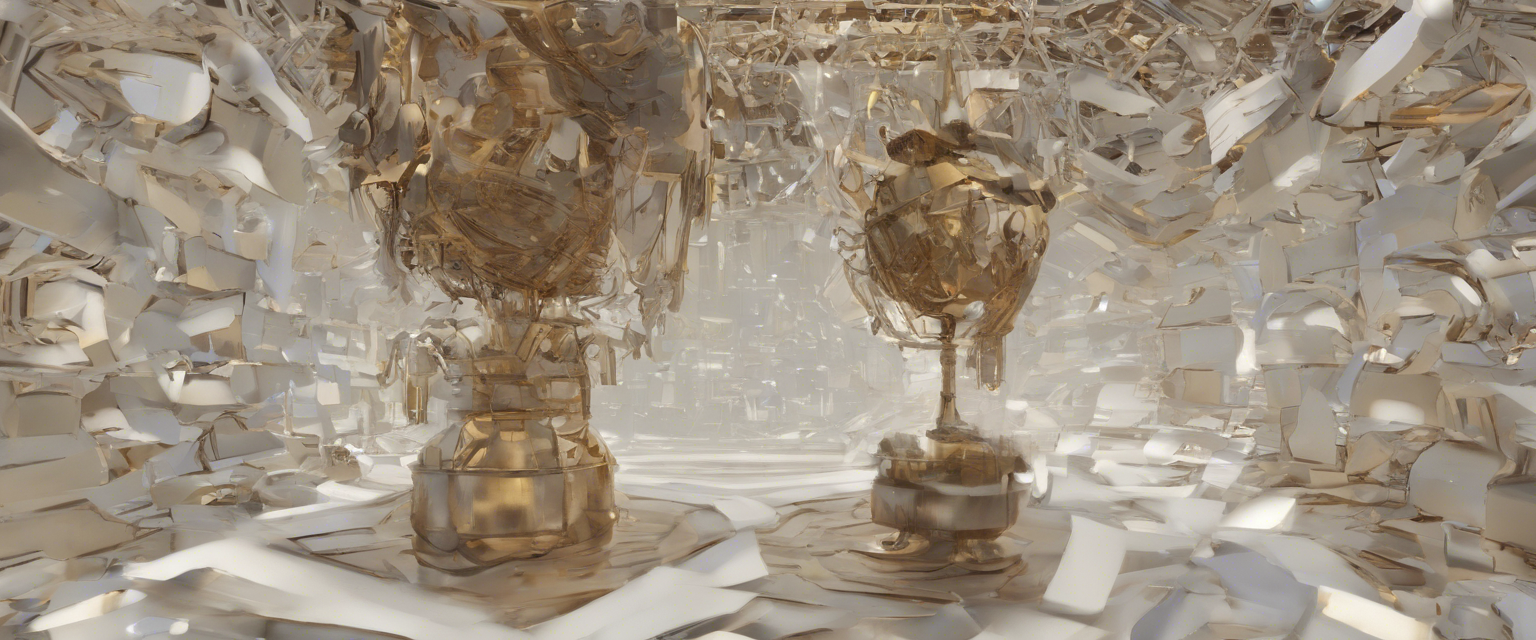
Folks, let’s talk AI. No, not the sentient kind that’s about to steal our jobs and write better screenplays than us (though, honestly, who isn’t a little worried about that?). I’m talking about the nuts and bolts, the silicon and software, the stuff that makes this whole AI revolution possible.
Benchmarking the Brainiacs
When it comes to training AI, one name reigns supreme: Nvidia. And the latest MLPerf benchmarks, the so-called “Olympics of machine learning,” only solidify their dominance.
Think of MLPerf as the ultimate AI proving ground. It’s where tech titans like Nvidia, Intel, and Google flex their computational muscles on standardized tasks. These tests are designed to offer a clear, objective comparison of AI training performance across different systems.
Now, this time around, MLPerf has added two particularly intriguing tests to the mix:
Fine-tuning large language models (LLMs): This is all about taking an existing, already-trained AI model (think something like ChatGPT) and giving it a specialized education. You’re essentially fine-tuning its knowledge for a specific task, like summarizing legal documents or writing marketing copy.
Graph neural networks: Imagine a vast network of interconnected points—think of a social network or a complex scientific database. Graph neural networks are adept at understanding these intricate relationships, and they’re used in everything from fraud detection to literature analysis.
Nvidia Flexes Its Muscles (Again)
So, how did things shake out in these new tests? Well, surprise, surprise, Nvidia stole the show. One system, a behemoth boasting 11,616 of Nvidia’s H100 GPUs (the most ever used in an MLPerf run), crushed all nine benchmarks, setting five new records in the process, including those two new tests.
Here’s where it gets really interesting. Nvidia achieved near-perfect “linear scaling.” In simpler terms, doubling the number of GPUs pretty much halved the training time. That might sound obvious, but in the world of high-performance computing, it’s no small feat.
Fun Fact: Getting twice the performance by simply doubling the hardware isn’t always a given. In fact, as you scale up these massive AI systems, you often encounter communication bottlenecks and other gremlins that can limit efficiency.
But Nvidia isn’t just about brute-force hardware. They’ve also been busy tweaking their software, and those improvements are paying off big time. They’ve squeezed even more performance out of their existing Hopper architecture by making clever optimizations to memory management, power usage, and even the way data flows between GPUs.
Trivia Time: One of the tricks Nvidia used is called “flash attention.” This ingenious algorithm speeds up transformer networks (the backbone of LLMs) by cleverly reducing the amount of data that needs to be written to memory. It’s like finding a shortcut through a traffic jam.
The Future of AI: A Scaling Showdown
But don’t think Nvidia’s going to have the AI playground all to themselves. Intel and AMD are both gearing up to challenge their supremacy with new chips of their own. We’re likely to see a three-way battle for the AI training crown in the coming years.
Why is this scaling battle so crucial? Because the AI systems of the future aren’t going to be trained in your average server room. We’re talking about “AI factories” packed with hundreds of thousands, even millions, of GPUs, churning through mind-boggling amounts of data. Getting those systems to scale efficiently is going to be the key to unlocking the next generation of AI breakthroughs.
This isn’t just about bragging rights; it’s about pushing the boundaries of what’s possible with artificial intelligence. And for those of us who are both excited and terrified by the possibilities, it’s a competition worth watching.
Comments
Post a Comment