AI's Next Frontier: Solving Real-World Problems
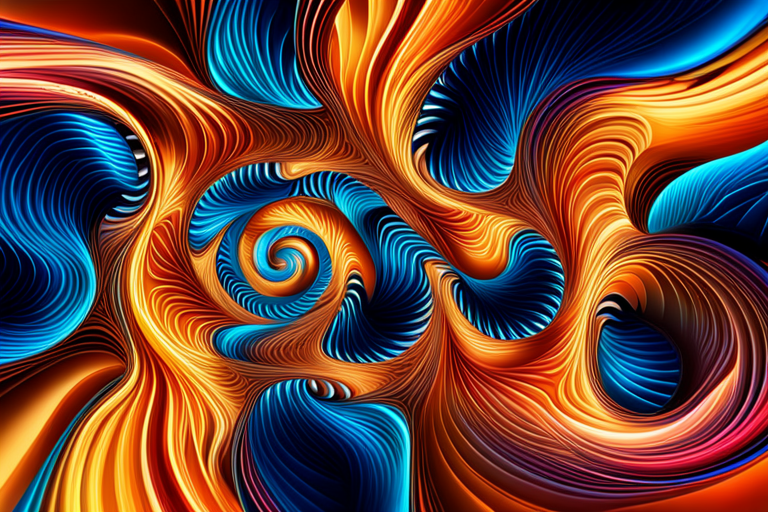
The Next Frontier in AI: From Research Papers to Real-World Solutions
The landscape of artificial intelligence is ever-evolving, transitioning from theoretical research to tangible, practical applications. While the invention of the Transformer model in 2017 marked a significant leap forward, the true potential of AI lies not in churning out research papers, but in tackling and solving complex, real-world problems. This shift in focus, recognized early on by organizations like OpenAI and DeepMind, signals a new era where AI transcends academic boundaries and becomes an integral part of our daily lives.
The Evolution of AI Development
To appreciate this evolution, it’s crucial to understand the distinct phases of AI development:
- Pre-2012: The Prehistory: While this categorization might make some AI pioneers bristle, the reality is that pre-2012 AI models struggled to deliver accurate and reliable results.
- 2012-2017: The Rise of Deep Learning: Deep learning emerged as a dominant force, propelling AI from a niche field to a technological cornerstone. Google Brain, a hotbed of innovation during this period, fostered a culture of “bottom-up” research, where brilliant minds explored open-ended questions, leading to groundbreaking discoveries like the Transformer and diffusion models.
- 2017-Present: The Age of the Transformer: The Transformer, a versatile model capable of handling diverse machine learning tasks, ushered in a new paradigm. This period emphasizes solving large-scale problems by focusing dedicated teams, akin to the Apollo project, on specific real-world challenges like robotics and natural language processing.
The Power of Compute and the Role of Data
The transition from GPT-2 to ChatGPT highlights another critical aspect of AI development: the interplay between model intelligence and its accessibility to consumers. While GPT-3 possessed impressive capabilities, it wasn’t until it was packaged into the user-friendly ChatGPT that it captivated the public’s imagination.
This leads to a crucial debate in AI: the significance of compute power and data in enhancing model performance. While some argue that we are reaching the limits of what increased compute can achieve, the reality is more nuanced. While simply scaling up existing models might yield diminishing returns, a new frontier of model improvement is emerging.
Rethinking Model Improvement
Instead of just feeding models more data, the future lies in enabling models to learn and improve autonomously. Imagine an AI tasked with solving complex math problems. Instead of relying solely on a dataset of solved problems, this AI would have access to a theorem-proving environment. It could experiment, learn from its mistakes, and refine its problem-solving strategies, much like a human mathematician.
This approach, incorporating elements of reinforcement learning and simulation, represents a paradigm shift in AI development. By providing models with the tools to explore and learn independently, we unlock a new level of intelligence that surpasses the limitations of static datasets.
The Future Landscape: From Model Providers to Application Builders
This shift also has profound implications for the AI industry. While a handful of well-resourced companies might dominate the foundational model layer, the real innovation will occur at the application layer. Companies like Adept, focused on building AI agents capable of handling complex workflows, exemplify this trend.
This transition from model-centric to application-centric AI development will reshape the industry in several ways:
- Vertical Integration: Building successful AI agents requires tight integration between the model, the user interface, and the specific use case. Companies like Adept, focused on building AI agents capable of handling complex workflows, exemplify this trend.
- The Rise of AI Services: As AI becomes increasingly embedded in enterprise workflows, the demand for implementation and support services will skyrocket. While this sector might experience significant growth in the short term, the long-term winners will be companies that can transform bespoke solutions into repeatable, scalable products.
- Redefining Business Models: The notion of “pricing per work” often touted in the context of AI, assumes a level of task commoditization that doesn’t align with the reality of knowledge work. AI agents, functioning as co-pilots and collaborators, will augment human capabilities, leading to new pricing models that reflect the value of enhanced creativity and productivity.
Navigating the Challenges: Regulation, Ethics, and the Future of Work
As AI becomes increasingly powerful, we must address critical challenges:
- Regulatory Landscape: The potential for regulatory capture in the AI industry is a real concern. Policymakers, often lacking a deep understanding of the technology, might inadvertently stifle innovation by favoring established players. Fostering a regulatory environment that encourages competition and open-source development is paramount.
- Open vs. Closed Systems: The debate surrounding open versus closed AI systems will intensify. While open systems foster innovation and accessibility, concerns about the potential misuse of powerful AI, particularly in the context of AGI, cannot be ignored. Finding the right balance between openness and control will be crucial for ensuring the responsible development of AI.
- The Future of Work: The narrative of AI replacing human jobs is simplistic and misleading. Instead of viewing AI as a job-stealing threat, we should focus on designing AI systems that augment human capabilities, empowering workers to be more creative, productive, and fulfilled.
The next decade of AI will be defined by our ability to move beyond hype cycles and focus on building AI systems that address real-world problems, empower human potential, and shape a future where humans and AI thrive together.
Comments
Post a Comment