Breaking Barriers in AI: EX Tropic Revolutionizes Semiconductor Industry with Stochastic Physics
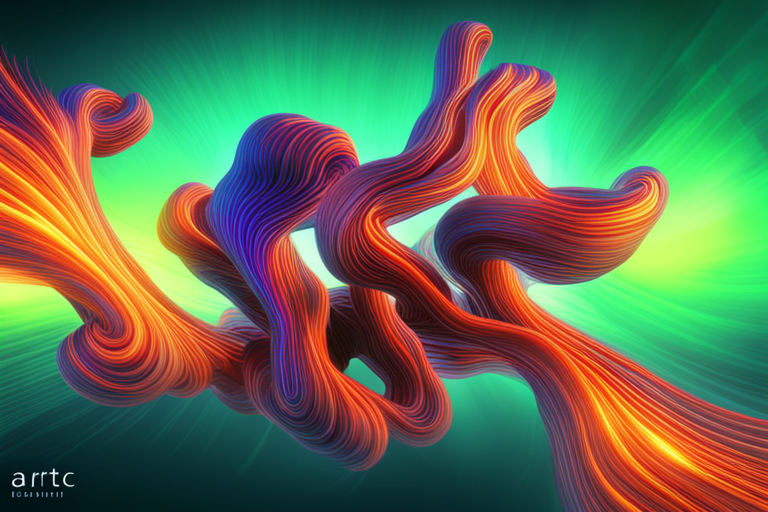
Stochastic Physics: The Next Frontier in AI and Semiconductor Industry
In a world where silicon-based transistors are nearing their limits, where the demand for artificial intelligence (AI) is skyrocketing, and where nuclear power plants are being suggested as a solution to power vast data centers, two pioneers are proposing a different path forward. Enter Gom Verun and Trevor McCort of EX Tropic, who are introducing a new approach to the AI and semiconductor industry with their full-stack, physics-based computing paradigm. This innovative solution, harnessing stochastic physics directly, is poised to redefine the landscape of AI and technology at large.
The Challenge: Moore's Wall
The foundation of this groundbreaking approach lies in a problem that the tech industry has been grappling with: the end of Moore's Law. This law, named after Gordon Moore, co-founder of Intel, has held true for decades, predicting that the number of transistors on a chip doubles approximately every two years, leading to exponential growth in computing power. However, as transistors shrink smaller and smaller, we're approaching the limits of their reliability - a barrier now referred to as 'Moore's Wall'.
Why is this happening? The answer lies in the very fabric of matter. At minuscule scales, matter experiences thermal fluctuations, causing electrons to sometimes get stuck or fail to cross a transistor. This essentially means that as we scale down, transistors become stochastic, or random, in nature.
The Solution: Stochastic Physics in AI
What if, instead of fighting this randomness, we embraced it? AI algorithms already incorporate stochasticity, adding probabilistic behavior through techniques like dropout or softmax sampling in large neural networks. Verun and McCort's proposition is simple yet revolutionary: implement AI algorithms in accordance with the stochastic physics of the world.
Their creation, EX Tropic, is a full-stack physics-based computing paradigm focused on AI. This new approach uses the stochastic physics of electrons directly to instantiate probabilistic machine learning. The technology is based on superconductors, which Verun and McCort refer to as the most efficient "neurons" in the universe. But these are not digital neurons - they're analog, stochastic circuits, signaling a departure from the digital norm.
This fundamentally not only necessitates the reinvention of the basic building block of computing but the entire stack that goes with it. It's a tremendous undertaking, but one that Verun and McCort believe is crucial for the continued evolution of AI and technology as a whole.
The Result: Efficient Sampling
The primary function of these devices is to accelerate sampling. In probabilistic models on a computer, you often need to draw samples from a distribution to calculate an average or similar statistic. On digital computers, this process is energy-intensive, especially for complex distributions, due to the need for complex, high-power transistor circuits to generate pseudo-randomness.
EX Tropic's solution circumvents this by leveraging the inherent randomness in stochastic physics, making sampling significantly more efficient. This approach could revolutionize how we handle AI and large data sets, potentially leading to more powerful, efficient, and sustainable tech solutions.
For more detailed insights into the world of AI and this innovative approach, you can explore mindburst.ai. If you're interested in other technology-related topics, peruse through Aharonoff Tech Tales.
Comments
Post a Comment