Revolutionizing Online Commerce: Enhancing AI Recommendation Engines for Superior User Experience
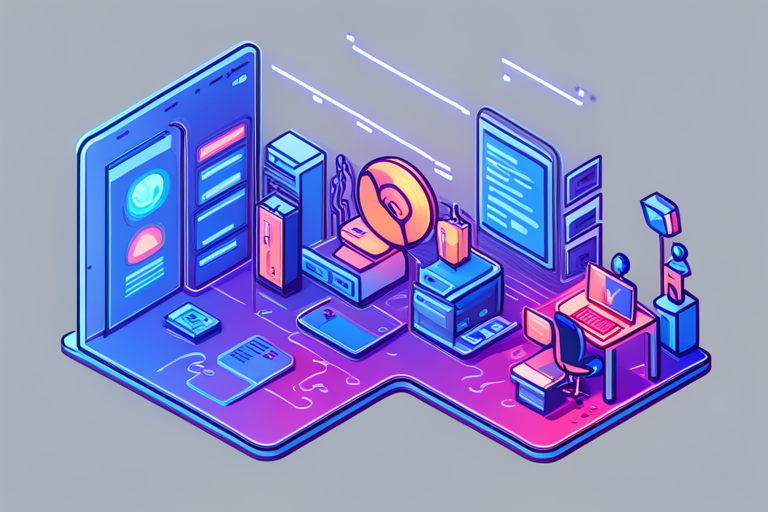
The Fascinating Journey for Superior AI Recommendation Engines
Imagine this: you've just finished watching a riveting TV series on your favorite streaming platform. As the credits roll, you're left in a state of emotional turmoil, wondering how any other series could possibly fill the void left by that gripping finale. Lo and behold, your streaming service steps in, saying, "You liked this TV series, so we think you'll like this one!" Or picture yourself browsing an online fashion store. As you're admiring a pink linen skirt, a pop-up suggests, "Consider these cream espadrilles to go with it!" This is the power of AI recommendation engines, the silent commerce drivers, the invisible salespeople who know exactly what your heart desires. But their integration doesn't fit neatly into existing machine-learning toolchains. So, how do they work? And more importantly, how can they be improved?
The Anatomy of AI Recommendation Engines
AI-based recommendation engines are not a new concept. They've been around for a while, with YouTube's uncanny ability to suggest videos you might like next, and TikTok's addictive algorithm that knows what you'd love to see. These recommendation systems are key players in the world of online commerce, driving sales by showing customers the products they're most likely to purchase.
Fun Fact: The global AI recommendation engine market was valued at USD 1.16 billion in 2020 and is projected to reach USD 20.16 billion by 2028, growing at a CAGR of 40.2% from 2021 to 2028.
The power of these engines lies in their ability to analyze vast amounts of data and use machine learning algorithms to identify patterns and predict user behavior. However, integrating them into existing machine-learning toolchains is not a straightforward task, and there is always room for improvement.
The Journey for Improvement
These recommendation engines are already incredibly effective, but they're not perfect. There's always room for improvement, and that's where the quest for better AI recommendation engines comes in. Unlocking AI's true potential can lead to more accurate, personalized, and efficient recommendations.
-
Accuracy: Higher accuracy in recommendations means a better user experience and higher conversion rates. This can be achieved by refining the machine learning algorithms and incorporating more relevant data.
-
Personalization: Users are more likely to engage with recommendations that are tailored to their preferences and behaviors. Advanced AI techniques like deep learning can be used to create more personalized recommendations.
-
Efficiency: Efficiency in this context refers to the speed and computational resources required to generate recommendations. Using more efficient algorithms can reduce the time taken to generate recommendations and lower the computational resources required.
In the B2B SaaS realm, for example, better AI recommendation engines can help businesses identify potential clients, recommend products or services based on past purchases, and predict future purchasing behaviors.
Trivia: Netflix claims that its recommendation system saves it $1 billion per year by reducing the number of cancelled subscriptions.
The final word on this quest isn't just about refining existing technologies; it's about exploring new frontiers in AI and machine learning. The future of recommendation engines might lie in emerging fields like generative AI, as discussed in the article Unlocking the Power of Generative AI.
As we venture deeper into this fascinating journey, we can only expect AI recommendation engines to become more accurate, more personalized, and more efficient. The future is indeed exciting, and I can't wait to see what it holds.
Comments
Post a Comment