AI Server Cost Analysis: Memory Challenges Impacting Market Growth and Efficiency
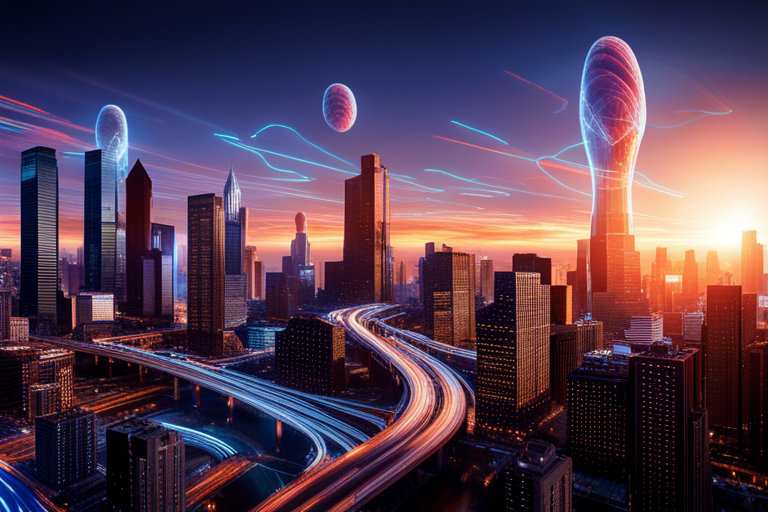
As we witness the rise of artificial intelligence applications and the development of more sophisticated AI models, the demand for powerful data centers has increased exponentially. This growth has led to a frantic race among businesses to build out AI-focused data centers. As a result, the markets have experienced a wild ride, with numerous companies experiencing significant growth. But amidst this rush, one crucial aspect of server cost analysis seems to have been overlooked: the role of memory.
The Memory Bottleneck
The most significant cost in running AI servers is memory, and this is turning out to be the biggest loser in the AI server cost analysis. Micron, a leading manufacturer of memory solutions, is experiencing a downturn in its AI-related performance, indicating that the market may not be well-prepared for the growing needs of AI applications.
Trivia: Did you know that Micron is among the top five semiconductor companies worldwide, known for producing NAND flash, DRAM, and NOR flash memory?
The Effects on the Market
As a result of the memory bottleneck, several companies have experienced fluctuating market performance. For instance:
-
Credo, a semiconductor company, experienced a 27% increase in the market in just one week. However, they don't reap many benefits from the AI server boom. As we previously discussed, they lost their only AI socket and have strong competition in the AEC and ACC space.
-
Vicor, a power management and delivery solutions provider, also saw a 30% increase in its market value. But we also revealed how they lost their position as the supplier to the Nvidia H100.
These examples highlight the market's potential misallocation of resources, as certain companies may not be well-positioned to capitalize on the AI server boom.
The Need for a Shift in Focus
To unlock AI's true potential, a shift in focus is required, with more emphasis placed on efficient memory solutions. This could involve exploring various types of memory technologies, such as:
- High-bandwidth memory (HBM)
- GDDR6 and GDDR6X
- Solid-state drives (SSDs) with NVMe interface
In addition, market players need to consider the role of software optimization in reducing memory requirements. By adopting techniques such as model pruning, quantization, and other optimization strategies, AI models can be made more efficient, potentially reducing the impact of the memory bottleneck.
As we move forward in the AI revolution, it's crucial that businesses, investors, and technology developers take a more nuanced approach to AI server cost analysis. By doing so, we can better allocate resources to address the pressing needs of AI applications and ensure the long-term success and sustainability of the AI ecosystem.
In the ever-evolving world of AI, memory is indeed the biggest loser, but it doesn't have to be that way. By acknowledging the challenges and addressing them head-on, we can pave the way for a more efficient and cost-effective future in AI server technology.
Comments
Post a Comment